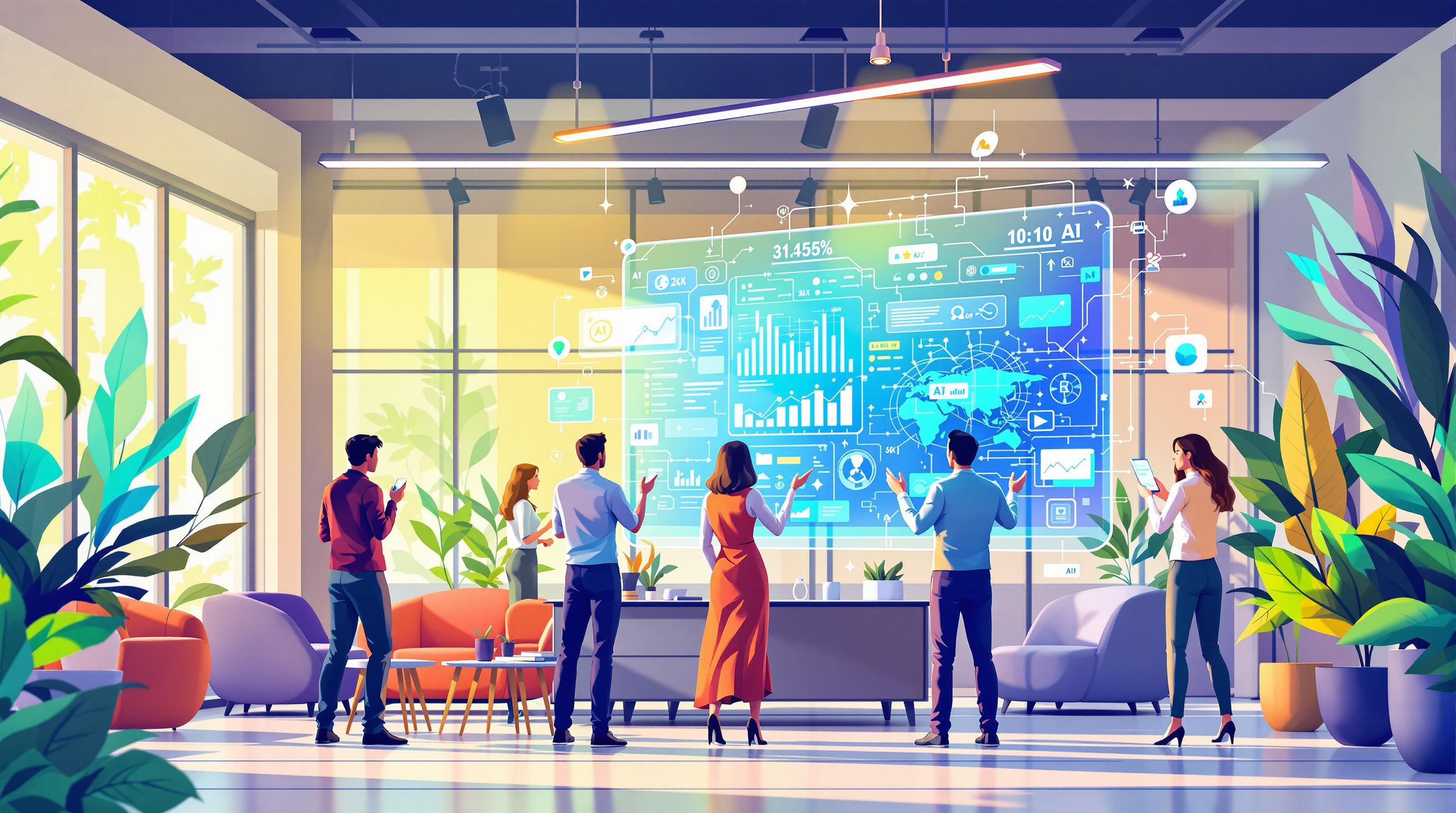
Back to Blogs
Implementing AI Agents at Scale: Strategies for Sustainable Enterprise Adoption
Technology
Implementing AI Agents at Scale: Strategies for Sustainable Enterprise Adoption
Scaling AI agents is critical for businesses aiming to improve efficiency, decision-making, and system performance. However, challenges like data integration, security concerns, and infrastructure limitations often slow progress. Here's what enterprises need to know:
-
Key Challenges:
- Integrating AI with existing systems and multiple data sources.
- Addressing security issues, with 62% of practitioners citing concerns over privacy and compliance.
- Scaling infrastructure to handle increased workloads without disruptions.
-
Solutions:
- Combine in-house expertise with external tools - 63% of executives favor this hybrid approach.
- Use integration platforms with features like real-time data processing, encryption, and auto-scaling.
- Focus on team training and clear governance frameworks to manage AI systems effectively.
-
Steps for Success:
- Start with small pilot programs and expand gradually.
- Monitor performance using metrics like adoption rates and system response times.
- Collaborate across departments to align AI goals with business objectives.
Introducing Azure AI Foundry Agent Service to scale your AI agents
Challenges Enterprises Face When Scaling AI Agents
When enterprises deploy AI agents across their operations, they often face a range of hurdles that can complicate the process. Tackling these issues is critical for both successful implementation and maintaining AI systems over time.
"Enterprises face growing integration complexity and organizational barriers in adopting AI-enabled SaaS applications." [1]
System Integration Struggles
Integrating AI systems into existing platforms is a major obstacle. Many integration tools aren't fully equipped to handle the intensive data demands of AI [1]. As a result, businesses often need to invest in additional solutions or develop custom integrations, which can slow down and complicate the rollout process.
On top of integration, enterprises must also navigate the complexities of security and compliance to ensure their AI systems are both effective and trustworthy.
Security and Compliance Concerns
Security is the top concern for enterprises, with 62% of practitioners and 53% of leaders highlighting issues like data privacy, regulatory compliance, and ethical AI use [1]. Companies need to ensure their AI systems meet industry standards while implementing strong security measures to protect sensitive information.
Infrastructure and Performance Hurdles
Scaling AI agents also brings infrastructure challenges. Enterprises need to address several performance-related issues:
- Resource Allocation: Managing computing resources efficiently is essential to prevent slowdowns and control costs.
- Scaling Infrastructure: As AI usage increases, systems must expand without disruptions.
- Maintaining Performance: Techniques like load balancing and auto-scaling are vital for consistent system performance [2].
"The survey highlights that as AI adoption grows, enterprises must rethink data integration, security, and accessibility to succeed." [1]
To overcome these challenges, 63% of executives are opting for a hybrid approach, blending in-house AI capabilities with third-party solutions [3]. This strategy allows organizations to maintain control while leveraging external expertise to address technical difficulties.
Resolving these infrastructure and security issues is essential for creating a scalable and dependable AI environment.
Preparing for AI Agent Deployment
Getting ready to deploy AI agents involves more than just flipping a switch. It takes thorough preparation across several areas. Did you know that 86% of enterprises need to upgrade their tech stack before they can fully embrace AI agents? [1].
Evaluating Technical Capabilities
Before diving into AI agent deployment, companies need to take a hard look at three key areas:
- Data integration: Do you have seamless access to the data your AI agents will need?
- Computing resources: Is your GPU or cloud infrastructure up to the task?
- Network infrastructure: Can your bandwidth handle the increased load?
After assessing these areas, it’s crucial to set up governance frameworks to guide the process.
Building an AI Governance Plan
A solid governance plan is essential. It should clearly define roles, ethical guidelines, and performance metrics to ensure the AI deployment aligns with your business goals. This framework keeps projects on track and prevents them from spiraling out of control.
But governance isn’t just about rules - it’s also about the people. Teams need the right skills and knowledge to manage these systems effectively.
Improving AI Knowledge Across Teams
As of now, 48% of enterprises are starting to use AI agents, while another 33% are actively exploring their options [3]. To support this momentum, organizations should focus on:
- Offering hands-on training with AI tools
- Encouraging collaboration between departments to share insights
- Keeping teams updated on the latest AI developments
The emphasis should be on practical, goal-oriented training. This helps teams not only maintain AI systems but also tackle the technical and organizational hurdles that come with deployment.
The secret to success? Address both technical readiness and team capability at the same time. This balanced approach lays the groundwork for scaling AI agent deployments effectively.
Steps for Scaling AI Agents in a Sustainable Way
Combining In-House and External AI Tools
A mix of internal development and third-party solutions works best for scaling AI effectively. In fact, 63% of executives have found success with this approach [3]. It keeps sensitive operations under control while taking advantage of external tools to save time and cut costs. This strategy helps organizations balance data security with the challenges of integrating new technologies.
Using Platforms for Scalable Integration
Integration platforms play a key role in handling the complexities of AI deployment, but they must meet specific data demands to be effective. When evaluating a platform, focus on these essential features:
Feature Category | Key Requirements |
---|---|
Data Management | Connectivity across multiple sources, Real-time processing with standardized formats |
Security | End-to-end encryption and detailed access controls |
Scalability | Auto-scaling capabilities and optimized load balancing |
Monitoring and Improving AI Performance
Keeping an eye on AI performance means focusing on workload distribution, scaling resources, and analyzing interactions for improvement. To achieve this, organizations should:
- Use dynamic workload balancing to maintain steady performance [2].
- Set up auto-scaling systems to respond to real-time demand [2].
- Review AI interactions regularly to spot areas for improvement [2].
The most effective companies scale AI step by step - starting small, analyzing results, and expanding based on performance data. This gradual approach ensures systems remain stable while continuously improving.
Tips for Long-Term Success with AI Agents
Once you've laid the groundwork for scaling AI agents, the next step is ensuring success over the long haul. This involves smart collaboration and thoughtful growth strategies.
Collaborating with Key Stakeholders
Working with the right stakeholders is essential to keep AI deployments on track and effective. When teams across different functions collaborate well, AI initiatives tend to perform better. Here are some areas where collaboration makes a big difference:
Area | Activities & Outcomes |
---|---|
Strategic Alignment | Host executive briefings and workshops → Align AI plans with overall business goals |
Technical Integration | Conduct team meetings and maintain clear documentation → Simplify deployment and avoid unnecessary technical issues |
Performance Monitoring | Schedule regular review sessions and gather feedback → Drive ongoing improvements and secure stakeholder support |
Starting Small and Expanding Gradually
With 86% of enterprises needing upgrades to their tech stacks for AI deployment [1], taking a step-by-step approach reduces risks and increases the likelihood of success. Here's how to do it:
- Strategic Pilot Programs: Begin with small pilot projects that clearly show value. Once these succeed, expand them to other departments or processes. In fact, 48% of enterprises are already succeeding with this method [3].
-
Performance Benchmarking: Use specific metrics to track progress at every stage. Examples include:
- Adoption rates among users
- Gains in process efficiency
- System response times
- Cost-effectiveness analyses
As you expand, it's critical to validate each step thoroughly. Strengthen security protocols as your AI systems grow to ensure they remain reliable and protected [1].
Examples of Successful AI Agent Deployments
Case Studies from Enterprises
IBM and Microsoft have shown how focusing on hybrid strategies and security can lead to effective AI implementation. For example, IBM streamlined its IT service desk operations by using a hybrid AI model, leading to notable efficiency improvements. On the other hand, Microsoft's enterprise-wide rollout emphasized the importance of strong integration and security measures [1] [3]. These examples highlight how different companies can tailor their approaches to successfully scale AI solutions.
Company | Focus Area | Results |
---|---|---|
IBM | IT Service Desk Automation | Improved efficiency with a hybrid AI model |
Microsoft | Enterprise-wide Integration | Achieved secure and scalable implementation |
Tray.ai | Data Integration | Delivered seamless enterprise connectivity |
Lessons from Industry Leaders
Top companies have identified several key factors for successful AI agent deployments, offering practical insights for others looking to implement similar initiatives.
"Optimizing AI agents isn't just about raw speed – it's about creating seamless, intelligent interactions that truly resonate with users." - Dr. Ava Chen, AI Performance Specialist [2]
Here are some shared traits of successful implementations:
- Infrastructure Preparation: Companies that evaluated and upgraded their technical capabilities before launching AI projects saw better outcomes. For instance, 86% of enterprises needed to enhance their tech stacks to support AI [1].
- Strong Data Integration: Organizations like Tray.ai highlight the importance of connecting diverse data sources effectively, ensuring smooth operations across systems [1].
- Financial Commitment: Many leading enterprises dedicate significant resources to AI, with 68% allocating at least $500,000 annually for development [1].
- Ongoing Performance Tracking: Real-time analytics and thorough benchmarks allow companies to identify and resolve potential issues early, maintaining AI success over time [2] [4].
Conclusion: Key Points for Scaling AI Agents
Adopting AI agents in enterprise settings comes with both opportunities and challenges. With 86% of enterprises requiring upgrades to their tech stacks, preparing infrastructure has become a top priority [1]. Tackling these challenges requires a clear focus on three main areas.
Scaling AI agents successfully requires significant investment. For example, 42% of enterprises plan to develop over 100 AI agent prototypes during their scaling efforts [1]. These efforts aim to address key issues like integration, security, and infrastructure.
Here are the three areas central to scaling AI agents:
-
Infrastructure and Integration
Enterprises need scalable integration platforms, especially since 48% report their current iPaaS products fall short for AI scaling [1]. This means assessing existing systems and planning necessary upgrades. -
Hybrid Implementation Strategy
A mix of in-house development and external solutions works best, with 63% of executives opting for this balanced approach [3]. This allows organizations to combine internal control with external expertise. -
Performance Optimization
For sustainable scaling, companies should track key performance metrics, such as:Focus Area Implementation Strategy Expected Outcome System Integration and Monitoring Unified platforms and regular benchmarks Smoother data flow and better efficiency Resource Management Auto-scaling and load balancing Better use of resources
With 48% of enterprises already adopting AI agents and another 33% actively looking into them [3], the focus must be on building strong foundations. This includes ensuring security, optimizing performance, and preparing for future scaling demands.
FAQs
What are the best 5 AI agent frameworks?
If you're scaling AI agents for enterprise use, these five frameworks stand out:
Framework | Key Strengths | Best Used For |
---|---|---|
Microsoft Autogen | Secure, Azure-ready | Large-scale enterprise deployments |
OpenAI Swarm | Multi-agent coordination, robust APIs | Complex distributed systems |
Vertex AI | MLOps, Google Cloud support | Data-intensive applications |
LangGraph | Flexible workflows, modular design | Custom agent development |
CrewAI | Team-focused, scalable | Collaborative AI implementations |
These tools simplify deployment while addressing essential needs like integration, governance, and real-time performance monitoring. For instance, LangGraph and Tray.ai are particularly effective at connecting multiple data sources, solving a common challenge for enterprises managing diverse systems.
"Enterprises are navigating an increasingly crowded sea of AI-enabled SaaS applications and confronting a perfect storm of integration complexity and organizational barriers." - Rich Waldron, Co-founder and CEO of Tray.ai [1]
When choosing a framework, focus on factors like integration compatibility, security, scalability, support, and cost. The right choice depends on your specific use case, technical requirements, and budget.
Keep in mind that selecting the right framework is just the beginning. Seamless integration and ongoing optimization will play a critical role in the success of scaling your AI agents.
Related Blogs
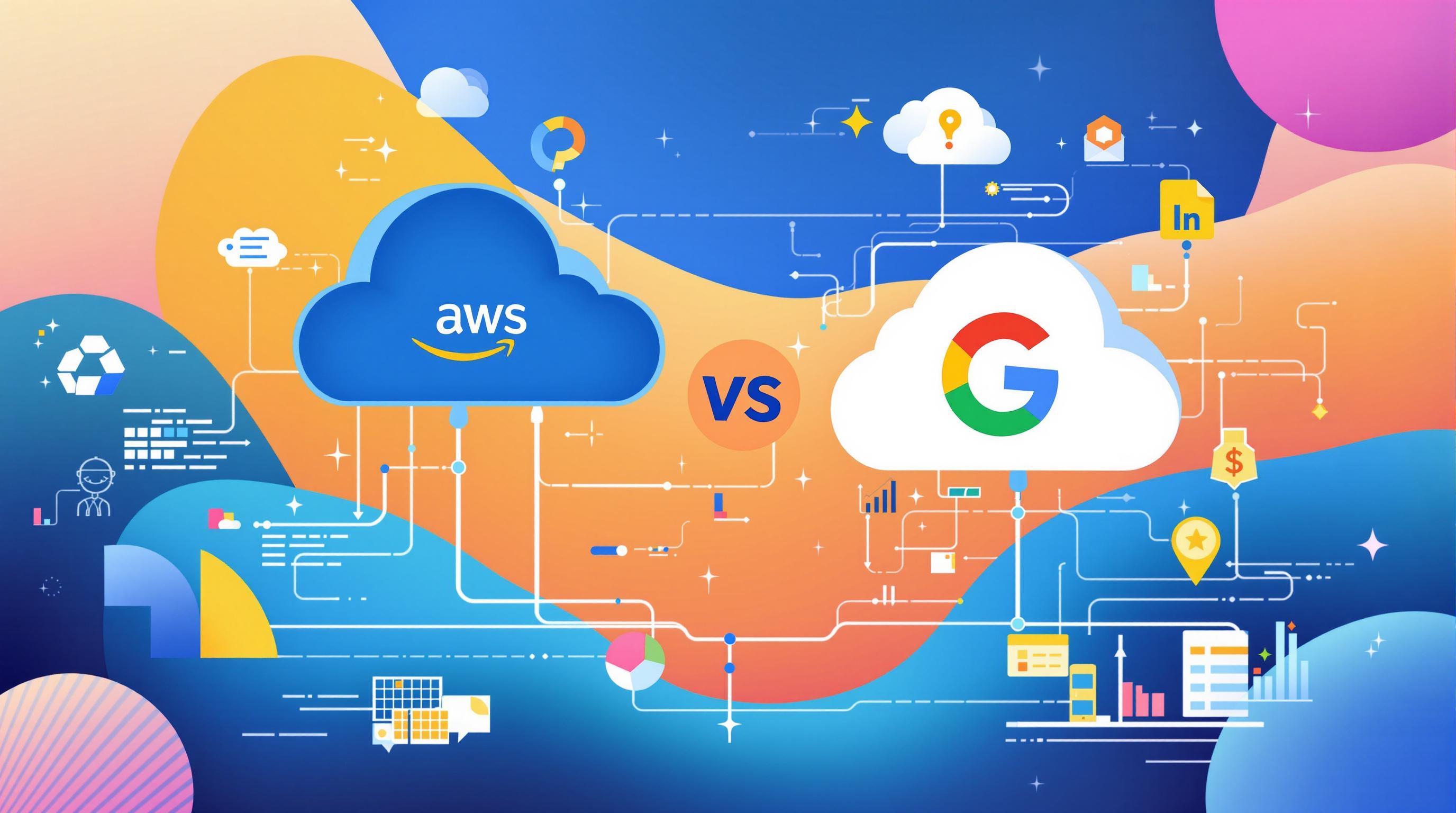
Technology
15 Jan 2025
Choosing the Right Cloud AI Platform: AWS vs. Google Cloud AI for Enterprise Applications
Evaluate AWS and Google Cloud AI to find the best fit for your enterprise's data strategy and AI needs, from integration to pricing models.
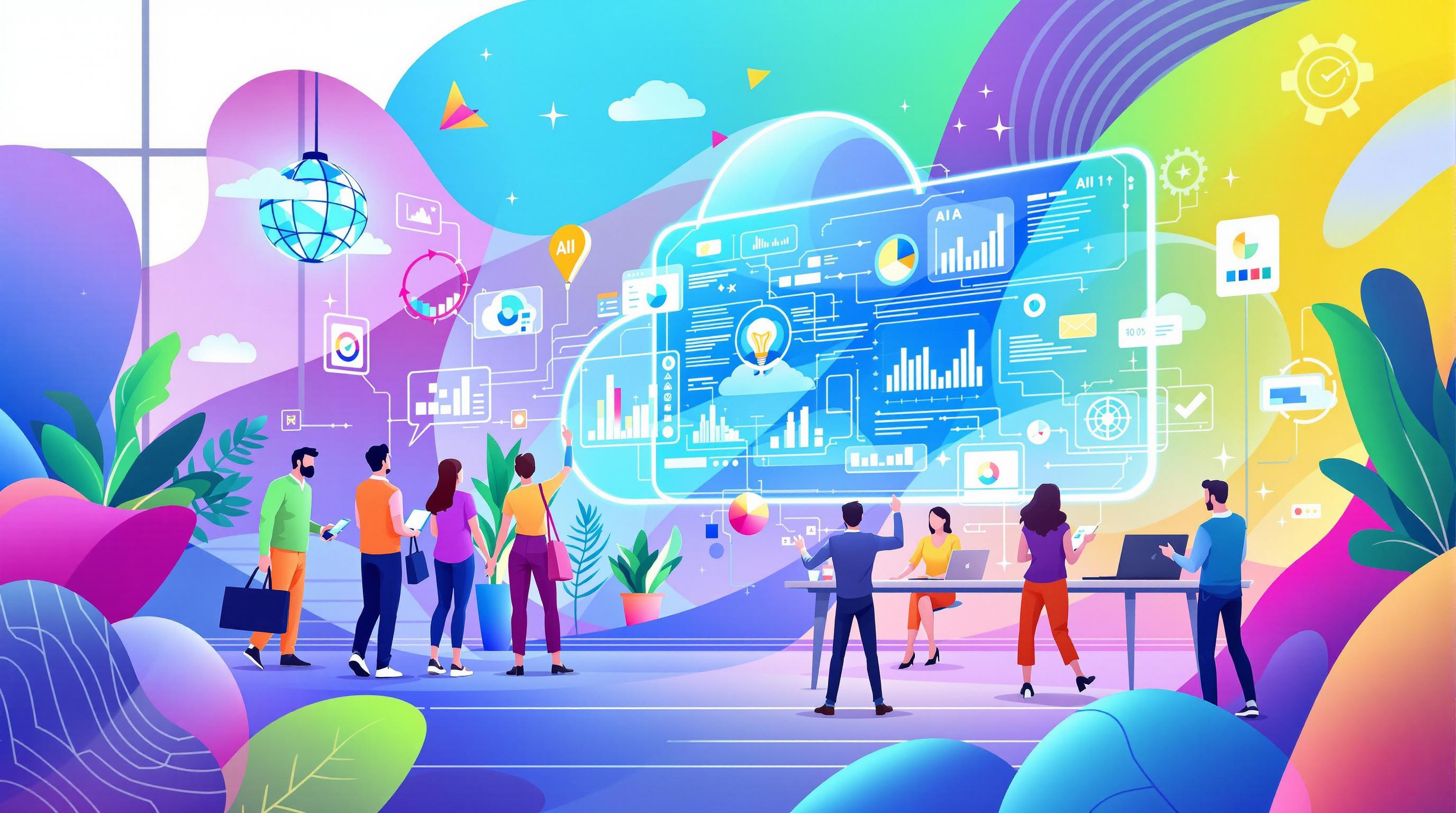
Technology
15 Jan 2025
How AI Agents Transform Enterprise Operations: Harnessing the Power of AI in the Cloud
Explore how AI agents are revolutionizing enterprise operations by enhancing efficiency, decision-making, and scalability through cloud technology.
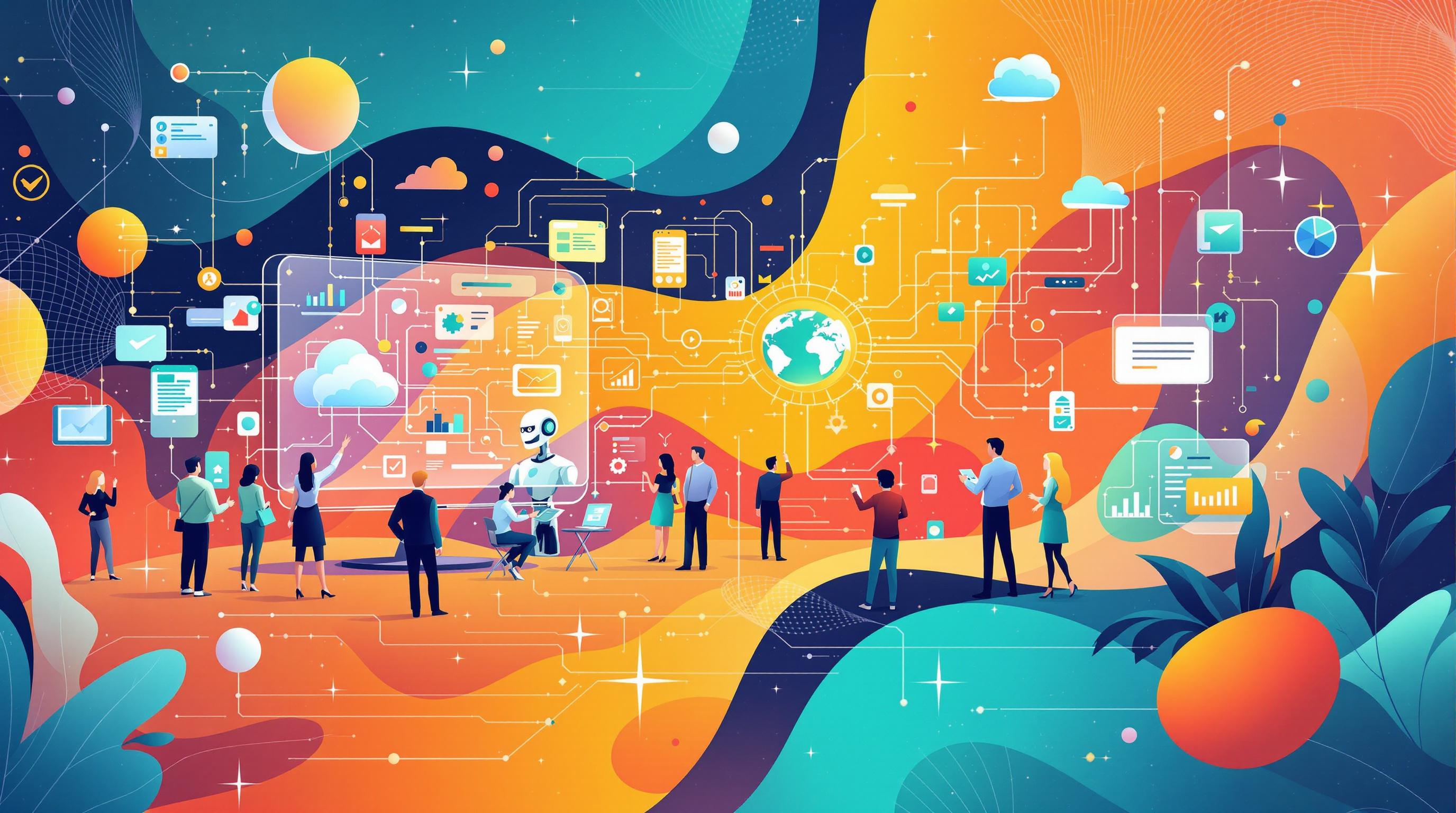
Technology
15 Jan 2025
AI Agents Beyond Chatbots: How Advanced Cloud Cognitive Services Drive Enterprise Growth
Explore how advanced AI agents powered by cloud cognitive services are driving enterprise growth through automation, data insights, and cost efficiency.